Case Study #1: Predict Accident involves using various data sources such as historical accident data, traffic patterns, weather conditions, and road infrastructure to create a predictive model that can forecast the likelihood of accidents occurring in a given area or time frame. This model can be used by traffic authorities, law enforcement agencies, and insurance companies to improve road safety and reduce the number of accidents. The model uses machine learning techniques to analyze and learn from the data, allowing it to identify patterns and make accurate predictions. By predicting accidents, authorities can take preventative measures such as improving road infrastructure, increasing police presence, and issuing alerts to drivers to reduce the likelihood of accidents occurring. This can help save lives, reduce injuries, and minimize the economic impact of accidents on society.
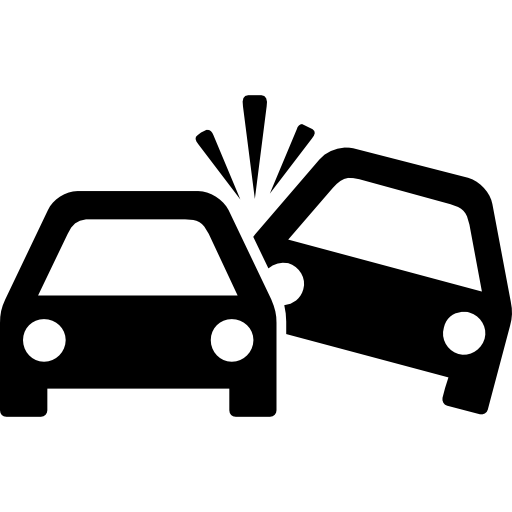
Case Study #2: Monitor Traffic flow and intensity are important factors to monitor in urban environments. The Department of Transportation are usually interested in a variety of things such as accidents, road coverage such as snow, crime, car breakdowns, speed of cars, traffic jams and number of pedestrians. Developing a deep learning model to monitor traffic from live-feed cameras involves using advanced computer vision techniques to analyze live video feeds and extract useful information about traffic patterns and conditions. The model uses deep learning algorithms to identify and classify various objects such as vehicles, pedestrians, and traffic signs, and can also analyze traffic flow, identify congestion, and detect accidents. By monitoring traffic in real-time, authorities can make informed decisions about traffic management, road infrastructure, and emergency response. This can help reduce traffic congestion, improve safety, and minimize the environmental impact of transportation. The deep learning model can be trained on large datasets of labeled images, allowing it to continuously improve its accuracy and performance over time.
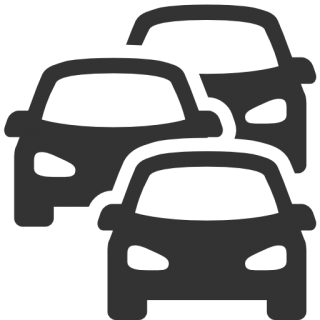
Case Study #3: Asset Inventory Management for railway (e.g., railway, milepost, crossing, signal, switch, etc.) requires comprehensive surveying and modern asset management. Besides inventory management of existing assets, large railway companies are also interested in 1) updating the existing inventory with new assets that are placed by the US Department of Transportation and 2) dropping the old assets from inventory that are removed from the railway by the US Department of Transportation. This is a very difficult task as large railway companies have lots of long railways (~over 10K mile) across the entire US. During the last few years, most of the large railway companies started to capture LiDAR data along the railway. Most of the large railway companies currently have a large number of technicians that go through 3D point clouds to detect their desired assets manually and label them as a point feature class. We used LiDAR technology to collect 3D point cloud data of a given area and advanced deep learning algorithms to analyze the data and identify various assets such as buildings, power lines, and other structures. The deep learning model uses neural networks to identify patterns in the LiDAR data and classify objects based on their shape, size, and location. By accurately identifying assets, businesses can make informed decisions about resource allocation, risk management, and cost optimization.
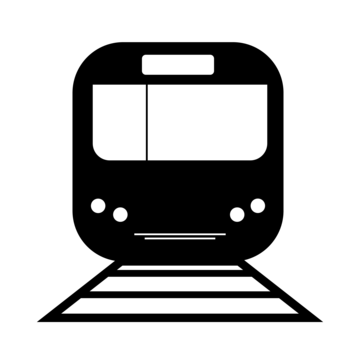