Case Study #1: Residential insurance companies rely heavily on data analytics to assess and manage risks associated with underwriting policies. One of the primary use cases is the evaluation of risk factors that could impact the likelihood of claims and their potential severity. By leveraging a wide range of data sources and advanced analytical techniques, insurers can make informed decisions, set accurate premiums, and reduce financial exposure. The process involves collecting and analyzing data on various risk factors, including the property’s location, age, and condition; local crime rates; historical weather patterns; and socio-economic demographics. Advanced machine learning models can then integrate these diverse data points to predict the likelihood of future claims, allowing insurance companies to fine-tune their risk assessment, optimize pricing strategies, and enhance overall operational efficiency.
By employing these data-driven insights, residential insurance companies can not only improve their risk management capabilities but also provide better value to their customers through personalized policy recommendations and proactive risk mitigation strategies.
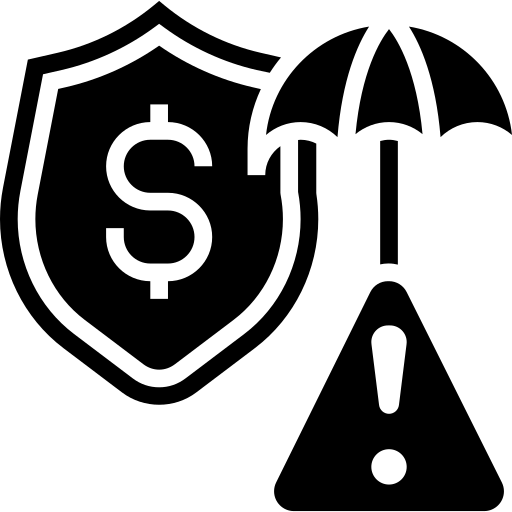