Case Study #1: In the competitive retail landscape, identifying optimal new store locations is crucial for business growth and success. Leveraging data science and socio-economic factors, businesses can make informed, strategic decisions about where to expand. This predictive approach involves analyzing a variety of socio-economic factors, including demographic data, income levels, employment rates, education levels, population density, and consumer spending patterns. By integrating these data points with advanced machine learning algorithms, businesses can uncover insights into potential market demand and profitability. The combination of socio-economic analysis and machine learning enables a data-driven approach to store placement, minimizing risks, and maximizing return on investment.
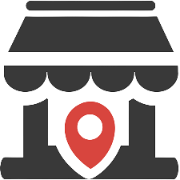
Case Study #2: Property valuation is a key analytic use case in real-estate, providing crucial insights for buyers, sellers, investors, and financial institutions. By utilizing data analytics, data scientists can integrate various factors such as historical sales data, neighborhood trends, climate-related risks, and social indicators to better understand market trends and price fluctuations. Climate insights, such as flood zones or natural disaster history, play a significant role in determining property values. Moreover, socio-economic factors like population growth, income levels, and employment rates can significantly impact property values. By analyzing these factors, data scientists can predict market demand and potential price appreciation. This data-driven approach not only enhances the efficiency and reliability of the valuation process but also aids in better decision-making and risk management. In essence, data analytics empowers real-estate professionals to make informed, strategic decisions, ultimately benefiting all stakeholders involved.
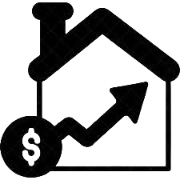