Case Study #1: Developing a Demand Forecasting Model for seeds in agriculture business involves using historical sales data, weather patterns, and other relevant factors to predict future demand for different types of seeds. The goal is to accurately forecast seed demand to ensure that the business has sufficient inventory to meet customer needs while avoiding overstocking, which can lead to inventory waste and financial losses. The demand forecasting model can be trained on large datasets of historical sales data, allowing it to learn from past trends and improve its accuracy over time. Additionally, other factors such as weather patterns, planting seasons, and market trends can be included in the model to make more accurate predictions. Demand forecasting can help seed manufacturers and distributors to plan their production and distribution strategies, ensuring that they can meet the demand in different regions and markets.
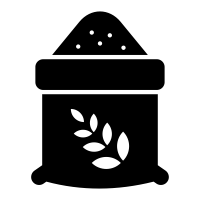
Case Study #2: Price Optimization Model can be used to optimize the price for agricultural seeds. The objective of the model is to maximize profit by setting the price of the seeds at the optimal level. The model takes into account the cost of production, the demand for the seeds, and the competition in the market. The cost of production includes the cost of materials, labor, and other expenses associated with growing and harvesting the seeds. The demand for the seeds is determined by factors such as the size of the market, the availability of substitutes, and the preferences of consumers. The competition in the market is determined by the number of suppliers and their pricing strategies. The model includes variables such as the price of the seeds, the quantity of seeds produced, and the revenue generated from sales. The objective function of the model is to maximize profit, which is calculated as the difference between revenue and cost. Sensitivity analysis can also be performed to determine how changes in the input variables affect the optimal price.
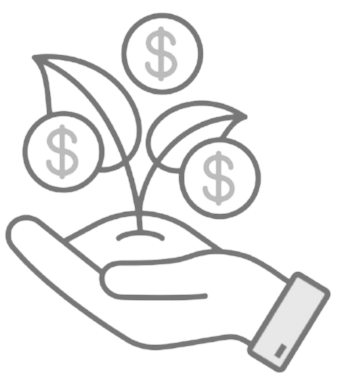
Case Study #3: Machine learning can be used to Recommend Seeds to farmers based on their location and weather conditions. This involves analyzing historical weather data and soil conditions to determine the best seed varieties that are suited for the specific region. Machine learning algorithms can also consider other factors such as crop yield and disease resistance to recommend the best seed varieties for farmers. These algorithms can also learn from past data and improve their recommendations over time. To implement this, farmers can input their location and weather data into the machine learning system. The system will then analyze the data and provide recommendations on the best seed varieties for their specific region. This can help farmers make informed decisions about their seed selection and increase their crop yield potential. Additionally, by using machine learning, farmers can save time and resources that would have been spent on manual seed selection and analysis.
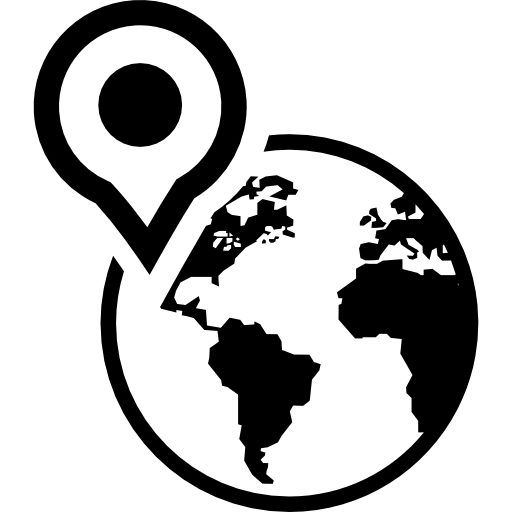
Case Study #4: To better understand the Product Life Cycle, it is important to evaluate the market demand for the specific type of seed. This includes analyzing the current trends and consumer preferences in the agricultural industry. Next, the seed’s performance and quality should be assessed. This involves analyzing the seed’s yield potential, resistance to diseases and pests, and overall durability. Additionally, the seed’s competition in the market should be evaluated. This includes analyzing the strength of the competition and their market share. Lastly, the seed’s innovation potential should be assessed. This includes analyzing the seed’s potential for genetic modification and other advancements in technology. By considering these factors, a prediction of the product life cycle for seeds in agriculture business can be made. This can help agricultural businesses make informed decisions about their seed offerings and marketing strategies.
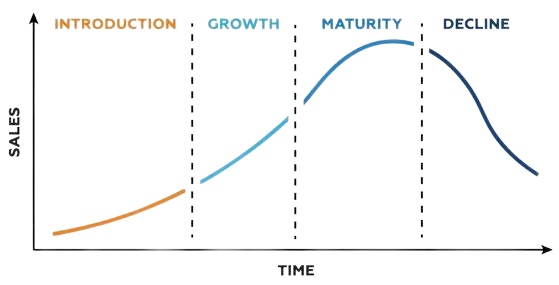
Case Study #5: Harvest Window Model involves training a model to predict the optimal time for harvesting based on weather conditions. The process involves collecting historical weather data and corn yield data from previous years. Features such as temperature, humidity, and precipitation are extracted from the weather data and used to train a machine learning model, such as a random forest or neural network. The model is trained to predict the corn yield based on the weather features, and the optimal time for harvesting is determined by identifying the point at which the yield begins to plateau or decline. This technique has the potential to greatly improve the efficiency and profitability of corn farming by allowing farmers to make informed decisions about when to harvest their crops.
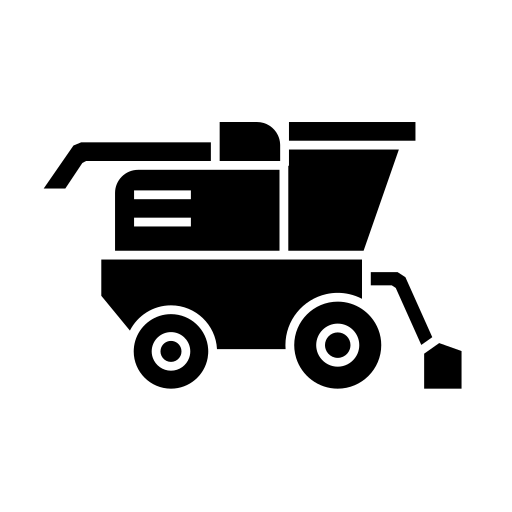
Case Study #6: Field Boundary Detection involves training a deep learning model to recognize the boundaries of agricultural fields from the aerial view captured by satellites. The process includes collecting high-quality satellite images and training a convolutional neural network (CNN) to identify the boundaries of fields. The CNN is trained on a dataset that includes labeled images of field boundaries and non-boundary regions. Once trained, the network can be used to automatically detect field boundaries in new satellite images. This technique has the potential to greatly improve the efficiency and accuracy of crop monitoring and management, as well as aid in land use planning and resource management.
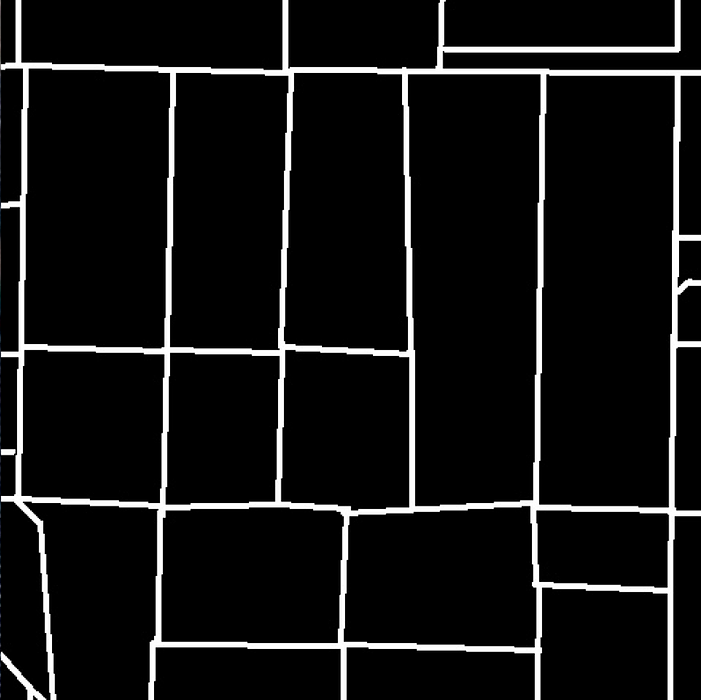
Case Study #7: Machine learning can be used to Predict the Yield of crops based on various factors such as seed type, soil quality, weather conditions, and farming practices. To predict yield using machine learning, historical data on crop yield, weather patterns, and soil quality are collected and analyzed. Machine learning algorithms then process this data to identify patterns and make predictions about future yields. These algorithms can also consider other factors such as crop management practices like irrigation, fertilization, and pest control. By analyzing all of these factors together, machine learning models can provide accurate yield predictions for farmers. Using machine learning to predict yield can help farmers make informed decisions about crop management and planning. By having a better understanding of their expected yield, farmers can optimize their use of resources, adjust their planting and harvesting schedules, and make informed decisions about crop rotation and management practices. Overall, using machine learning to predict yield can help farmers increase their crop yield potential and improve their profitability.
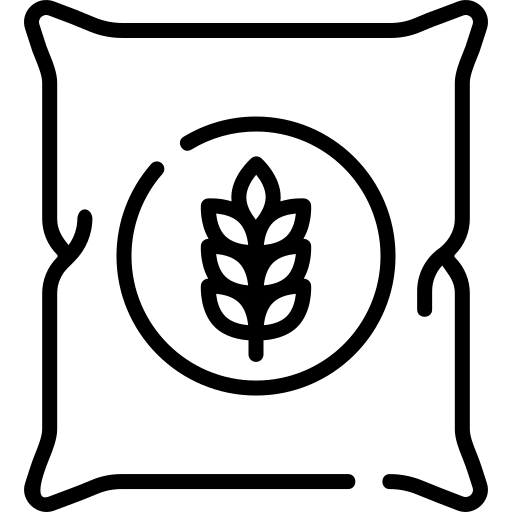
Case Study #8: SmartScape helps policymakers to evaluate the consequence of crop changes on various ecosystem services in agriculture landscapes. SmartScape 1) integrates multiple open-source software tools to build a user-friendly web client; 2) integrates a variety of spatial and temporal data and environmental models, into an interactive environment to allow stakeholders with various interests to build crop change scenarios; and 3) allows stakeholders to evaluate and identify suitable crop change policies by visualizing the tradeoffs among multiple ecosystem services in a timely manner. Assessment of participant feedback from potential users (group of stakeholders from Dane County as well as group of scientists who were experts in various disciplines, such as computer science, landscape ecology, agriculture, water quality, soil chemistry and climate change) reveals that this SmartScape™ is an effective tool to show the general consequences of various types of crop changes for developing effective crop changes policy.
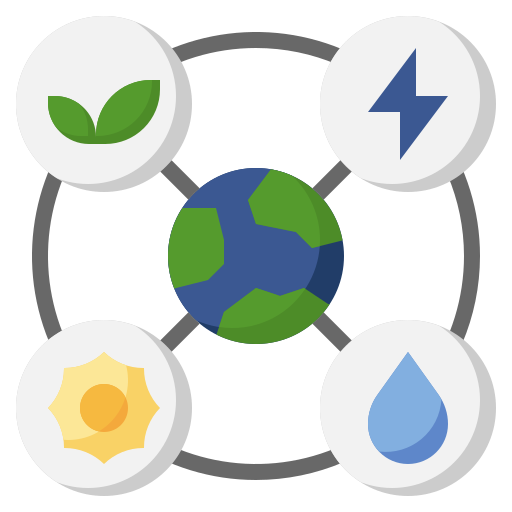